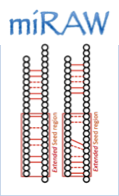
miRAW is a miRNA target prediction based on Deep Learning (DL) which, rather than incorporating any knowledge (such as seed regions), investigates the entire miRNA and 3’UTR mRNA nucleotides to learn a uninhibited set of feature descriptors related to the targeting process.
The trained model is based on more than 150,000 experimentally validated homo sapiens miRNA:gene targets cross referenced with different CLIP-Seq, CLASH and iPAR-CLIP datasets to obtain ∼20,000 validated miRNA:gene exact target sites.
Using this data, we implemented and trained a deep neural network—composed of autoencoders and a feed-forward network—able to automatically learn features describing miRNA-mRNA interactions and assess functionality. Predictions were then refined using information such as site location or site accessibility energy. In a comparison using independent datasets, our DL approach consistently outperformed existing prediction methods, recognizing the seed region as a common feature in the targeting process, but also identifying the role of pairings outside this region. Thermodynamic analysis also suggests that site accessibility plays a role in targeting but that it cannot be used as a sole indicator for functionality.